Artificial Intelligence Creates New Opportunities to Combat Fraud
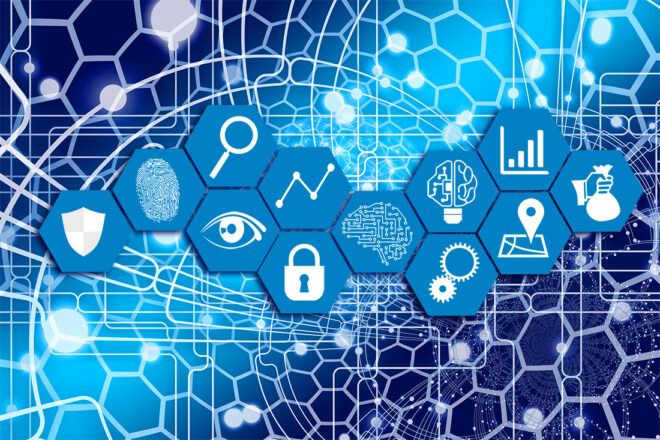
by Taka Ariga, U.S. Government Accountability Office (GAO)Chief Data Scientist and Director, GAO Innovation Lab; Johana Ayers, Managing Director, GAO Forensic Audits and Investigative Service (FAIS); Toni Gillich, GAO FAIS Assistant Director; Nick Weeks, GAO FAIS Senior Analyst; Scott Hiromoto, GAO Applied Research and Methods Senior Data Analyst; and Martin Skorczynski, Senior Data Scientist, GAO Innovation Lab
Combating fraudsters has long been a persistent challenge for governmental entities. While there are no precise figures, fraudulent activities drain billions of taxpayer dollars each year from vital programs. In today’s digitally-connected, information-driven world, the traditional approach to fraud detection work based on retrospective reviews by auditors is becoming increasingly ineffective. This “pay then chase” framework is resource-intensive, difficult to scale and fails to recapture a significant amount of known and suspected fraudulent transactions.
Fortunately, the proliferation of data alongside advances in computational capacities have ushered in the golden age for Artificial Intelligence (AI), where algorithms and models can reveal anomalous patterns, behaviors and relationships—with speed, at scale and in depth—that was not possible even a decade ago.
From Global Positioning System navigation to facial recognition, AI has fundamentally transformed every facet of our lives. Public sector organizations are similarly taking advantage of powerful algorithms to more prospectively detect and address red flags before they manifest into significant issues.
Importantly, AI does not replace professional judgments of experienced auditors in detecting potentially fraudulent activities. While AI can sift through large volumes of data with tremendous accuracy, human intelligence is still an essential element for determining context-specific, proportionate and nuanced actions stemming from algorithmic outputs. This symbiotic relationship means AI will assist Supreme Audit Institution (SAI) work and will change how that work is carried out—requiring different skills to harness AI’s capacity to drive effectiveness and efficiencies.
The U.S. Government Accountability Office (GAO) seeks to leverage the power of AI to improve government oversight and combat fraud, and GAO’s Innovation Lab, established in 2019 as part of the Agency’s new Science, Technology Assessment and Analytics unit, is driving AI experimentation across audit use cases (see Table 1 “AI Capabilities and Ongoing GAO Work”).
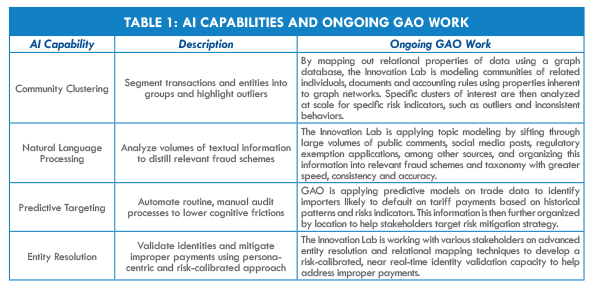
As progress is achieved, GAO aims to share success stories and lessons learned with SAIs and the broader accountability community. Concurrently, the Innovation Lab, in conjunction with all relevant stakeholders, is in the early stages of developing an AI oversight framework to help guide the development of AI solutions while adhering to overarching good practices and standards for auditors.
Table 1 also shows how GAO’s Innovation Lab is developing relevant fraud related analytical capabilities potentially forming the basis for future AI solutions. Each use case is designed to quickly identify hidden correlations, behaviors, relationships, patterns and anomalies that may be indicative of fraud risks.
Before pursuing AI analytical solutions to oversight and combating fraud, SAIs can benefit by considering important legal, societal, ethical and operational considerations that are especially relevant to AI. Moreover, SAIs can gain valuable insight from private and public sector organization experiences and lessons learned.
IMPORTANT CONSIDERATION WHEN USING AI
AI algorithms do not understand the difference between fraudulent and non-fraudulent transactions. Instead, these algorithms identify anomalies, such as unusual transactions between accounts. Human subject matter experts are still needed to analyze these anomalies to determine whether potential fraud exists.
To withstand scrutiny, audit institutions hoping to establish anti-fraud AI solutions may consider a set of guiding points, including how AI solutions are:
- Carefully Trained and Validated: Rigorously training and validating AI algorithms is required to minimize model errors. AI solutions that generate excessive false positives, such as by labeling too many legitimate transactions as potentially fraudulent, can overwhelm an organization and its ability to investigate potential fraud.Explainable,
- Logical and Reasonable: Explainable, well-defined and precisely documented AI algorithms are paramount. It is important to ensure modeled dependencies between variables are logical, underlying assumptions are reasonable, and model and algorithm outputs are expressed in plain language.
- Auditable: To conform to Generally Accepted Government Auditing Standards, thoroughly documenting applied AI techniques is critical. This includes parameters related to models and data sets used as well as the rationale for including any proprietary techniques, such as third party systems from external vendors.
- Governed: AI algorithm oversight is vital to ensuring consistent performance across different operating environments. It is imperative AI solutions, especially out-of-box algorithms, are free of negative impacts, such as unintentional discrimination again protected groups.
STEPS TO SUCCESSFULLY IMPLEMENT AI
Public and private sector organizations have identified several key steps for successful data analytics initiatives, including AI approaches:
Identify Objectives and Align Efforts: Identifying how specific program objectives may help meet organizational needs is recommended in the early stages of analytics program development.
Obtain Buy-in: Organizational support for data analytics and an appreciation of its ability to enhance goal achievement are essential. Creating a division responsible for developing analytical capability is one way to institutionalize knowledge.
Understand Current Capabilities: Initially, organizations can inventory existing resources to better understand capabilities and prioritize areas for improvement. Key resources include staff expertise, hardware and software as well as data sources and owners.
Include Users and Subject Matter Experts: Including the right subject matter experts in analytics projects can help inform model development and obtain buy-in from eventual model users.
Start Simple, Incrementally Build Capacity: Organizations may first seek to develop minimally viable solutions to meet goals. By identifying and obtaining quick and early successes, organizations can build a case to incrementally develop further capabilities, creating a foundation to implement more sophisticated AI solutions.
Transition to Operation: Once a minimally viable product is developed, moving it to a production environment is crucial. It is important to document updates to the AI algorithm.
SAI managers may consider several resources while exploring AI implementation. GAO’s Fraud Risk Framework identifies leading practices to help program managers combat financial and nonfinancial fraud. These leading practices include steps for using data-analytics activities to aid fraud detection, which can help establish a foundation for more sophisticated analytics, such as AI.
GAO’s “Highlights of a Forum: Data Analytics to Address Fraud and Improper Payments,” includes recommendations from the public and private sector on how to establish analytics programs. In particular, forum panelists provided suggestions on establishing and refining a data analytics program.
In 2018, GAO published the “AI Technology Assessment” identifying a range of AI-related opportunities, challenges and areas needed for future research and policymaker consideration.
The International Organization of Supreme Audit Institutions Working Group on Big Data helps facilitate knowledge sharing among SAIs on issues associated with data and data analytics.
Subject Matter Experts Interviewed
- Solon Angel, Founder, Chief Impact Officer, MindBridge Ai
- Jim Apger, Security Architect, Splunk
- Bart Baesens, Professor of Big Data & Analytics, Katholieke Universiteit Leuven, Belgium
- Justin Fessler, Artificial Intelligence Strategist, IBM Federal
- Robert Han, Vice President, Elder Research
- Bryan Jones, Owner and Principal Consultant, Strategy First Analytics
- Rachel Kirkham, Head of Data Analytics Research, United Kingdom National Audit Office (UKNAO)
- William Pratt, Data Scientist, UKNAO
- Wouter Verbeke, Associate Professor of Business Informatics and Data Analytics, Vrije Universiteit, Belgium